A/B testing (also known as split testing or bucket testing) is a methodology for comparing two versions of a webpage or app against each other to determine which one performs better.
A/B testing is essentially an experiment where two or more variants of a page are shown to users at random, and statistical analysis is used to determine which variation performs better for a given conversion goal.
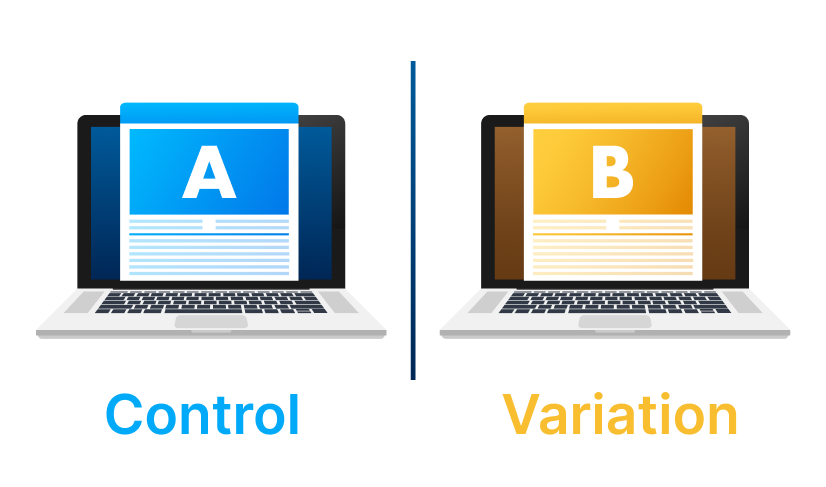
Running an A/B test that directly compares a variation against a current experience lets you ask focused questions about changes to your website or app and then collect data about the impact of that change.
Testing takes the guesswork out of website optimization and enables data-informed decisions that shift business conversations from “we think” to “we know.” By measuring the impact that changes have on your metrics, you can ensure that every change produces positive results.
How A/B testing works
In an A/B test, you take a webpage or app screen and modify it to create a second version of the same page. This change can be as simple as a single headline button, or be a complete redesign of the page. Then, half of your traffic is shown the original version of the page (known as control or A) and half are shown the modified version of the page (the variation or B).
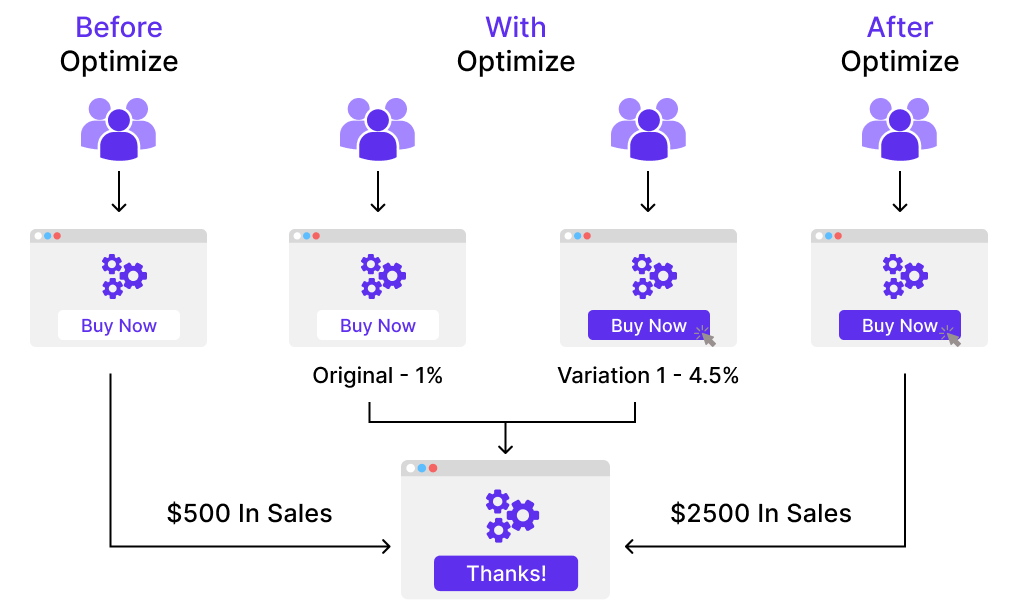
As visitors are served either the control or variation, their engagement with each experience is measured and collected in a dashboard and analyzed through a statistical engine. You can then determine whether changing the experience (variation or B) had a positive, negative or neutral effect against the baseline (control or A).
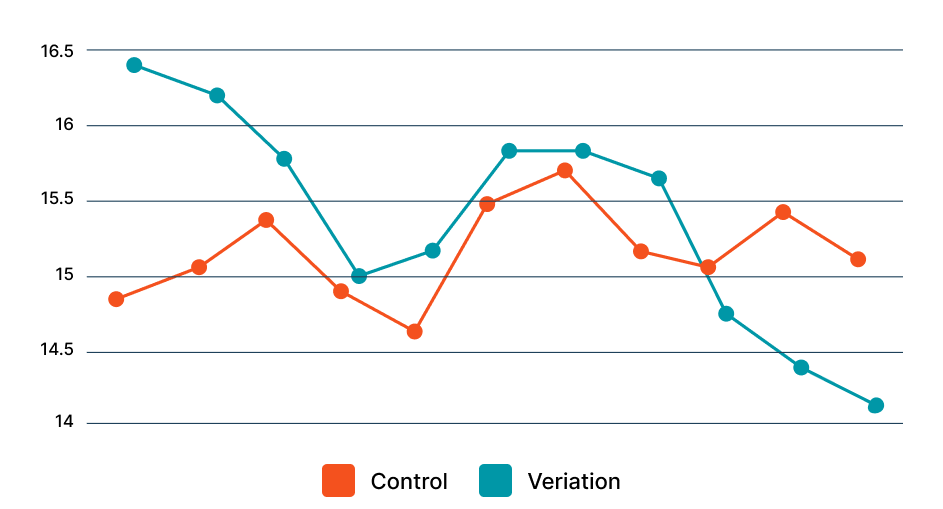
Why you should A/B test
A/B testing allows individuals, teams and companies to make careful changes to their user experiences while collecting data on the impact it makes. This allows them to construct hypotheses and to learn what elements and optimizations of their experiences impact user behaviour the most. In another way, they can be proven wrong—their opinion about the best experience for a given goal can be proven wrong through an A/B test.
More than just answering a one-off question or settling a disagreement, A/B testing can be used to continually improve a given experience or improve a single goal like conversion rate optimization (CRO) over time.
A B2B technology company may want to improve their sales lead quality and volume from campaign landing pages. In order to achieve that goal, the team would try A/B testing changes to the headline, subject line, form fields, call-to-action and overall layout of the page to optimize for reduced bounce rate, increased conversions and leads and improved click-through rate.
Testing one change at a time helps them pinpoint which changes had an effect on visitor behaviour, and which ones did not. Over time, they can combine the effect of multiple winning changes from experiments to demonstrate the measurable improvement of a new experience over the old one.
This method of introducing changes to a user experience also allows the experience to be optimized for a desired outcome and can make crucial steps in a marketing campaign more effective.
By testing ad copy, marketers can learn which versions attract more clicks. By testing the subsequent landing page, they can learn which layout converts visitors to customers best. The overall spend on a marketing campaign can actually be decreased if the elements of each step work as efficiently as possible to acquire new customers.
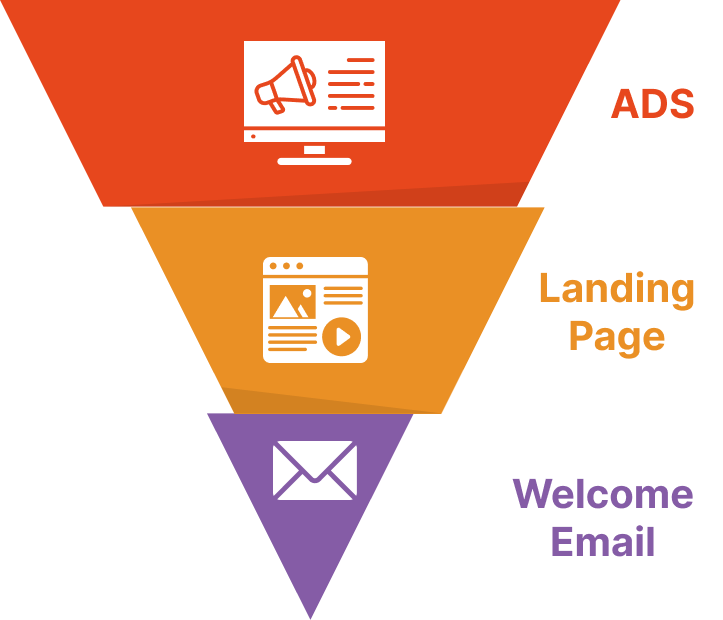
A/B testing can also be used by product developers and designers to demonstrate the impact of new features or changes to a user experience. Product onboarding, user engagement, modals and in-product experiences can all be optimized with A/B testing, as long as goals are clearly defined and you have a clear hypothesis.
A/B testing process
The following is an A/B testing framework you can use to start running tests:
Collect data:
Your analytics tool (for example Google Analytics) will often provide insight into where you can begin optimizing. It helps to begin with high traffic areas of your site or app to allow you to gather data faster. For conversion rate optimization, make sure to look for pages with high bounce or drop-off rates that can be improved. Also consult other sources like heatmaps, social media and surveys to find new areas for improvement.
Identify goals:
Your conversion goals are the metrics that you are using to determine whether the variation is more successful than the original version. Goals can be anything from clicking a button or link to product purchases.
Generate test hypothesis:
Once you’ve identified a goal, you can begin generating A/B testing ideas and test hypotheses for why you think they will be better than the current version. Once you have a list of ideas, prioritize them in terms of expected impact and difficulty of implementation.
Create different variations:
Using your A/B testing software (like Optimize Experiment), make the desired changes to an element of your website or mobile app. This might be changing the colour of a button, swapping the order of elements on the page template, hiding navigation elements, or something entirely custom. Many leading A/B testing tools have a visual editor that will make these changes easy. Make sure to test run your experiment to make sure the different versions as expected.
Run experiment:
Kick off your experiment and wait for visitors to participate! At this point, visitors to your site or app will be randomly assigned to either the control or variation of your experience. Their interaction with each experience is measured, counted and compared against the baseline to determine how each performs.
Wait for the test results:
Depending on how big your sample size (the target audience) is, it can take a while to achieve a satisfactory result. Good experiment results will tell you when the results are statistically significant and trustworthy. Otherwise, it would be hard to tell if your change truly made an impact.
Analyse results:
Once your experiment is complete, it’s time to analyse the results. Your A/B testing software will present the data from the experiment and show you the difference between how the two versions of your page performed, and whether there is a statistically significant difference. It is important to achieve statistically significant results, so you’re confident in the outcome of the test.
If your variation is a winner, congratulations 🎉🎉🎉! See if you can apply learnings from the experiment on other pages of your site, and continue iterating on the experiment to improve your results. If your experiment generates a negative result or no result, don’t worry. Use the experiment as a learning experience and generate new hypothesis that you can test.
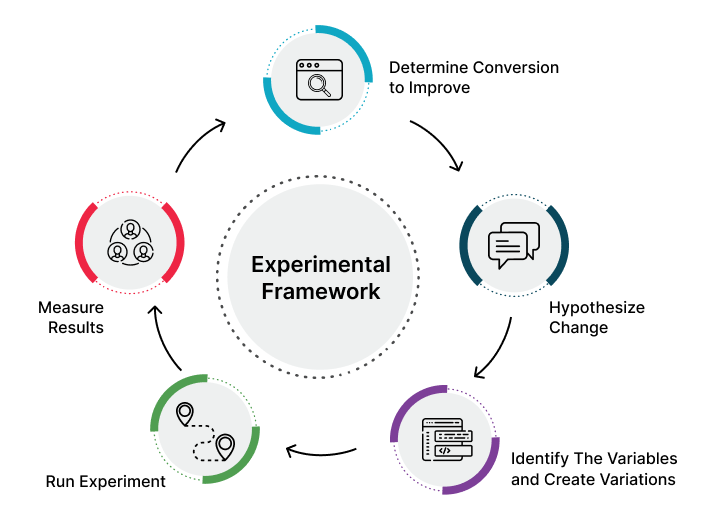
Whatever your experiment’s outcome, use your experience to inform future tests and continually iterate on optimizing your app or site’s experience.